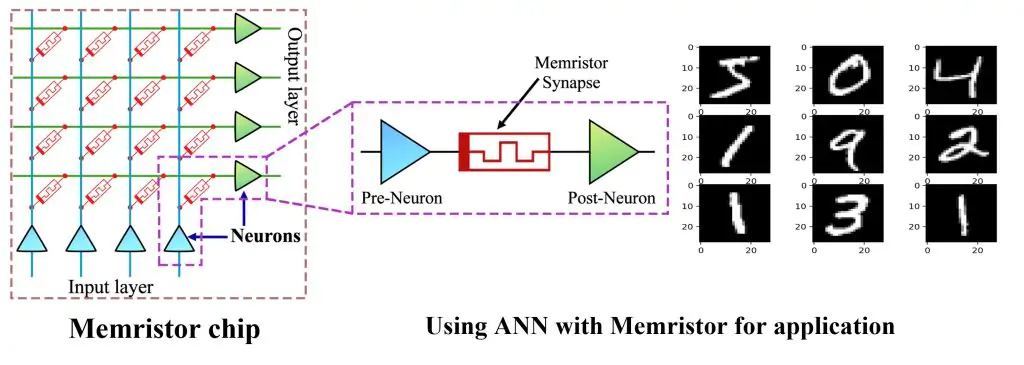
The project is implemented with the goal of successfully manufacturing memories that store data through different states of resistance values, known as resistive memory. The outstanding feature of this memory is the ability to change the storage state quickly according to the received voltage signal. This is an important feature that makes it possible to simulate the behavior of biological synapses. On this basis, the team will build an artificial neural network with resistive memory where neurons connect and store their communication information. Create an artificial neural network capable of storing distributed information instead of having to transmit it to external memory DRAM or Flash.
With the goal of basic, application-oriented research, the group conducts research with three specific goals:
• Successfully develop material systems applied to resistive memory, combined with theoretical calculations to evaluate the mechanism and control the operation of components effectively.
• Fabricate a memory chip structure with an integration density from 16 to 4096 memory cells and fabricate an electronic chip circuit with a memory chip acting as an artificial synapse.
• Develop algorithms and deploy them to be applied to recognize images, writing, faces… based on the in-memory computing architecture of the manufactured memory components.