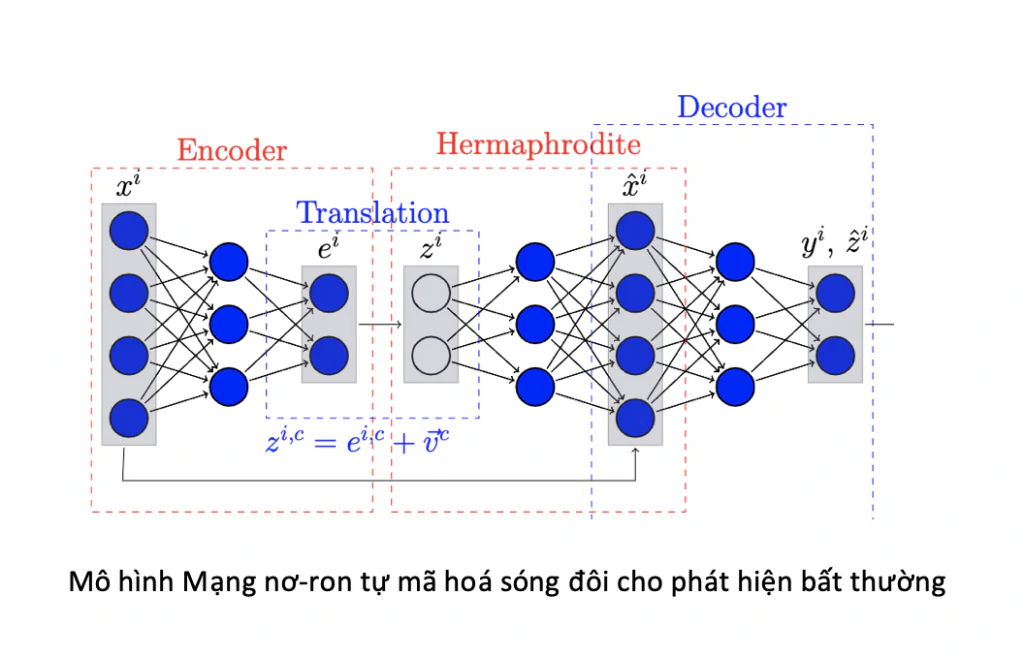
The goal of the project is to develop Separable Autoencoder neural network models for anomaly detection. The proposed models contribute to improving the accuracy of anomaly detection methods in cases where labeled data is scarce and many types of complex anomalies exist. Next, the project aims to apply the proposed solutions to solve a number of important real-life problems including information security and video surveillance.
Main tasks of the project
● Develop a novel self-training Autoencoder model that separates abnormal data from normal data in the hidden representation space.
● Develop a Twin Autoencoder network model with a deterministic separable hidden representation and learn to reconstruct the hidden representation using a twin architecture.
● Proposing a new federated learning model based on the Autoencoder model for anomaly detection in IoTs networks.
● Develop a memory augmented Autoencoder model to detect abnormalities in videos.
● Apply the proposed solutions to several real-world applications including cyber security and video surveillance.